Challenges
Faced by ATMS
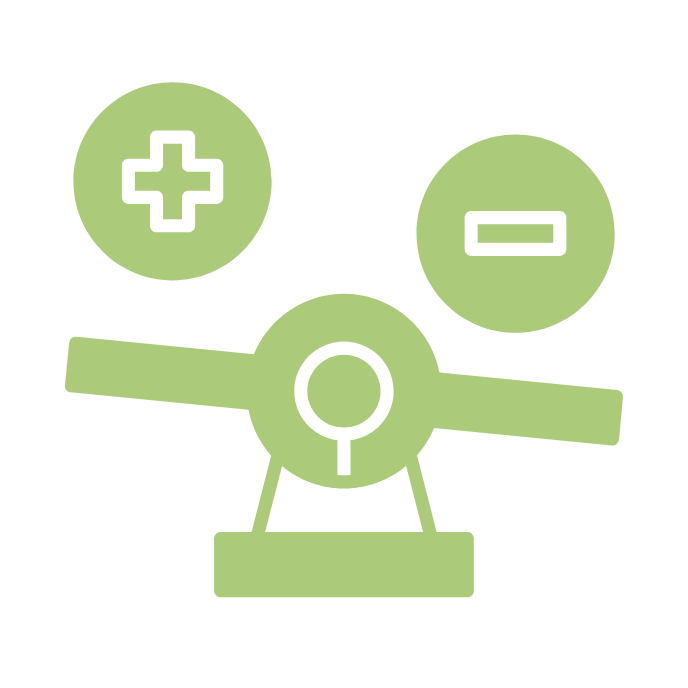
False Positives
ATMS can generate a high number of false positives, which means legitimate transactions are sometimes flagged as suspicious. This requires human review, which can be time-consuming. DAWN ATMS essential combination of curated data pipelines, operationalised machine learning models, and granular Complex Event Processing rule criterion significantly reduces false positives.
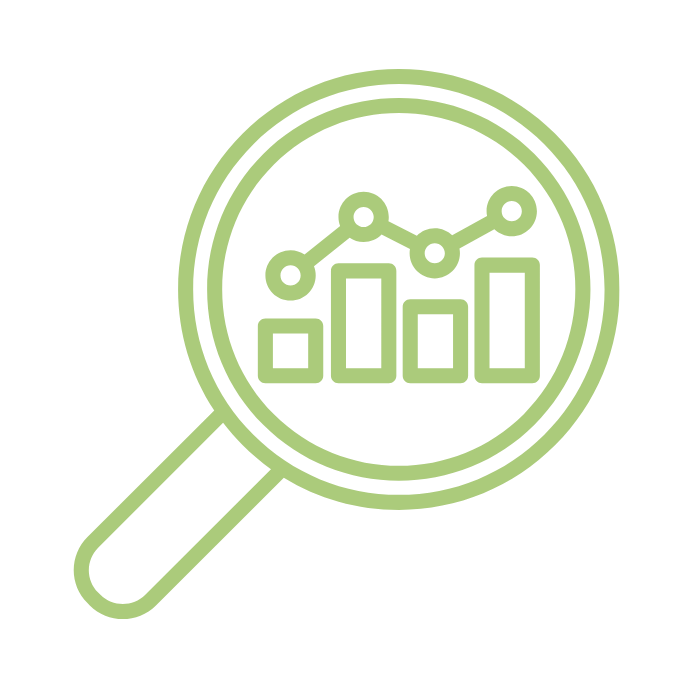
Link prediction
Using graph-based machine learning techniques to predict potential fraud by identifying likely connections between entities based on past behaviours and Context-aware detection - examining not just individual transactions but the broader context (e.g., relationships between accounts, devices, geolocations) to assess whether a transaction is consistent with normal behaviour or indicative of fraud significantly reduces instances of false positives.
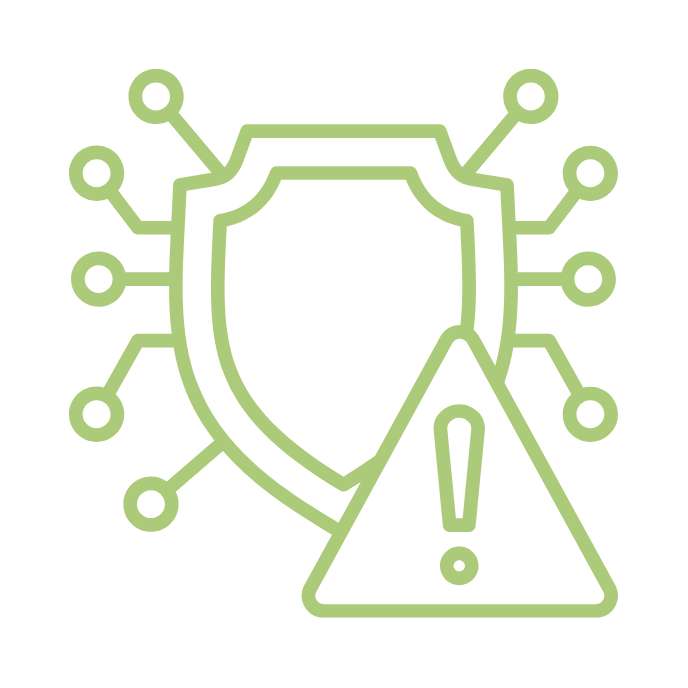
Evolving Threats
Criminals continuously evolve their methods to avoid detection, so the system must be regularly updated and refined to address new threats. The use of real-time threat feeds and machine learning counters the evolving nature of the threat environment.
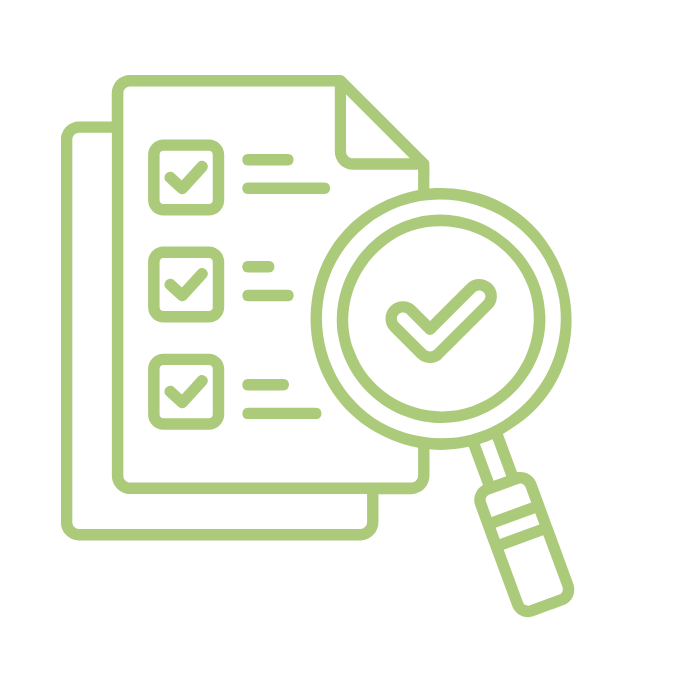
Data Quality
Poor or incomplete data can lead to missed suspicious activities or incorrect transaction flagging, reducing the system's effectiveness. DAWN ATMS’s ability to ingest and normalise data from a wide variety of data sources significantly offsets the risk of missed suspicious events or incorrect transaction flagging.