Framing Fraud Detection
Discover more about
Framing Fraud Detection through Gartner’s® Fraud Framework
Gartner® highlights the need for a layered fraud prevention approach to combat fraudsters and syndicates targeting enterprises.
This approach breaks down into five distinct layers, each focusing on different aspects of system security.
Layer 1
Is endpoint-centric, utilising technologies
like secure browsing and transaction-
signing devices to protect user interactions
with enterprise systems.
Layer 2
Monitors session navigation patterns to detect abnormal behaviour.
Layer 3
Is user- and account-centric within specific channels, analysing individual behaviour and transactions.
Layer 4 & 5
Broaden the scope by analysing behaviour across multiple channels and conducting entity link analysis to identify relationships between users, accounts, and other entities to detect organised fraud.
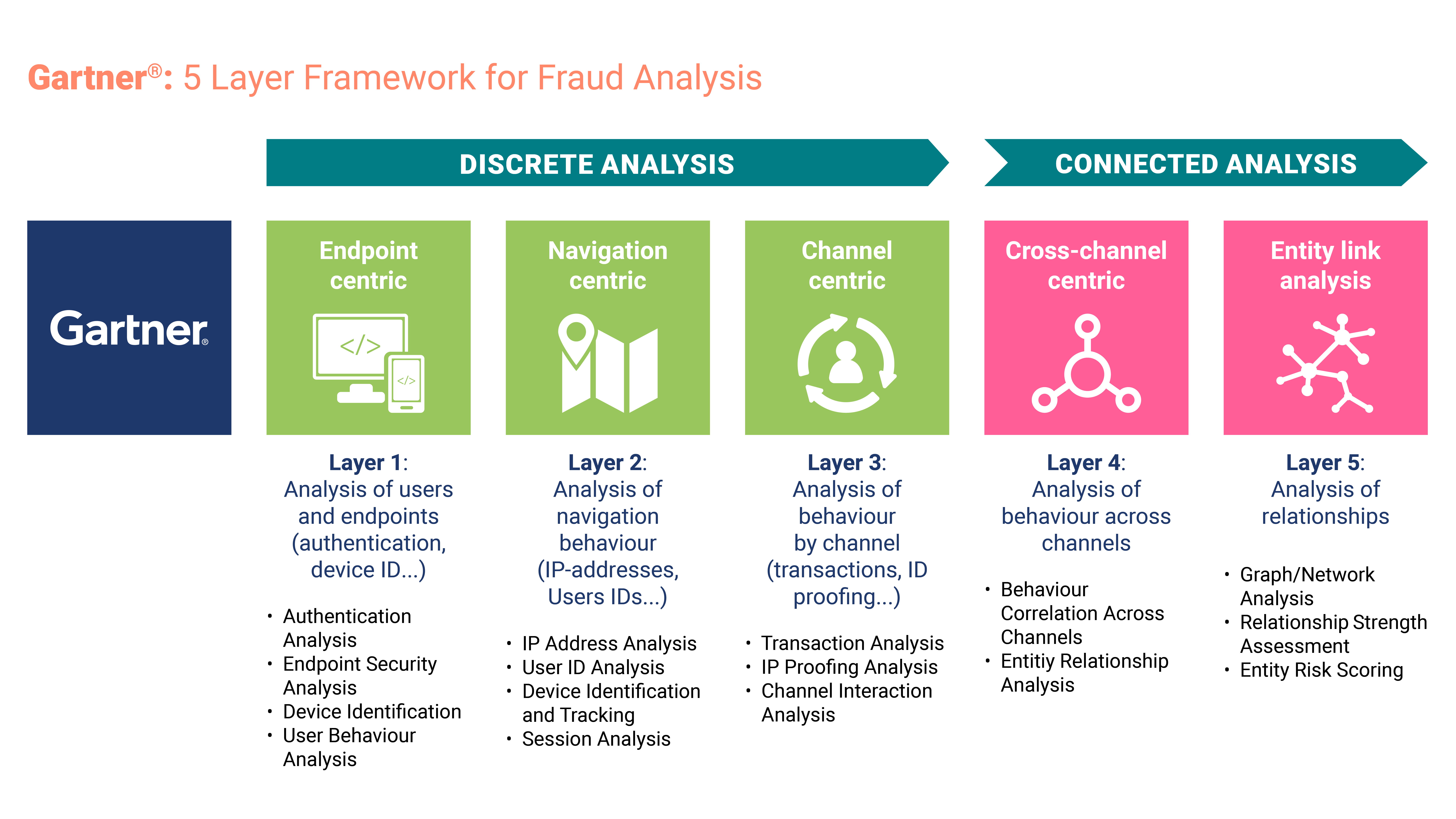
In a world where complexity breeds vulnerabilities, the true power of Risk X DAWN ATMS lies in its ability to connect the dots—leveraging graph technology to reveal hidden relationships and combat sophisticated fraud across diverse financial channels.
Why Use Dawn
Risk X DTMS is powered by LOCSTAT® LIGHTWEAVER’s strengths in both discrete and connected analysis align with this layered model.
Discrete analysis, which the earlier layers can handle, focuses on specific user behaviour and transactional anomalies.
However, DAWN ATMS’s real strength lies in connected analysis, which becomes essential in Layers 4 and 5, where multi-channel and entity link analysis occurs. Only graph-database-technology can effectively analyse and visualise relationships between multiple entities, a feature integral to identifying complex fraud patterns across connected networks.
This is especially relevant for African financial services, where financial systems often involve multiple channels (such as mobile wallets, banking apps, and agent networks) to serve unbanked and under-served populations. Locstat’s® graph-based platform enables financial institutions to handle this complexity, providing robust fraud detection by analysing relationships and patterns that static and layer-specific discrete systems cannot manage.